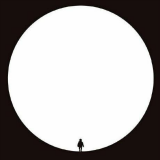
Blog of Disquiet
Technology. Philosophy. Writing.
Seneca wrote 124 letters to his friend Lucilius. They are published in his famous Letters from a Stoic. All are rather short but contain an immense amount of (Stoic) wisdom. I don’t want to talk about all of these letters, but focus on the very first one.
In it, Seneca talks about how much of our life is already behind us, already in death’s hand. It’s about living a meaningful and the revelation that “the largest portion of our life passes while we are doing ill, a goodly share while we are doing nothing, and the whole while we are doing that which is not to the purpose.
As everything in life programming comes with ups and downs. There are days when work is flowing and there are other days you are barely able to write a single line of code. Writing code professionally is an endeavour where celebration and frustration are never far apart. How can you best deal with this? What techniques help to soften these up and downs? One thing that helps me is way older than programming itself: the ancient philosophy of stoicism.
I love Python. Every time I write code I prefer it to be in Python. So, naturally, when I write a web service my #1 tool of choice is Django. This was also true as my girlfriend asked me a while ago if I couldn’t create as simple meal planner for her. Nothing fancy, just a simple view where we could organize our meals.
In times of stay-at-home orders it was rather easy to find time for projects like this.